The Security Implications of Working with AI Models
ByJulian Gette
Workast publisher
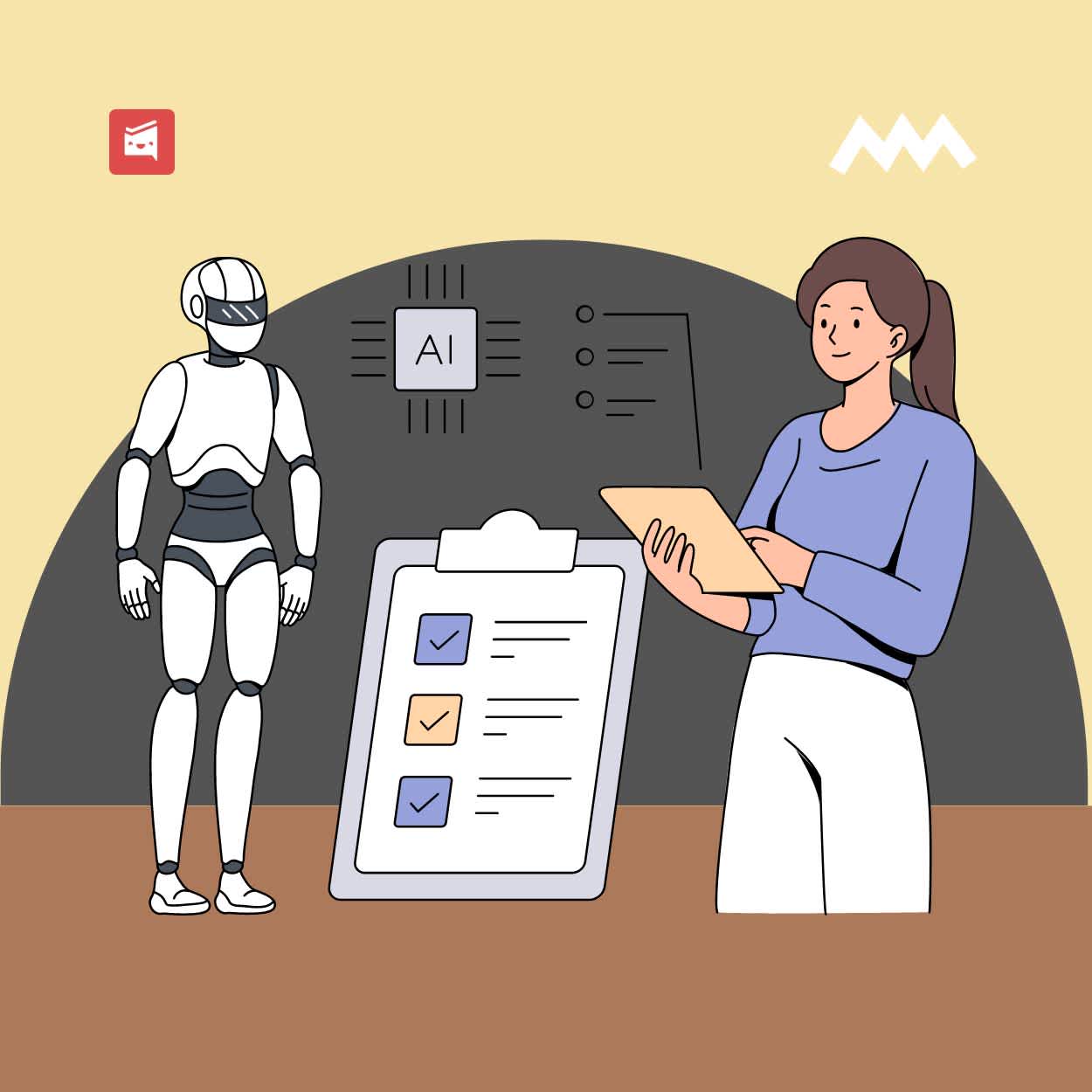
Workast publisher
Artificial intelligence (AI) models, including machine learning and deep learning algorithms, have become integral to various industries, from healthcare to finance to transportation. While these models offer significant benefits, they also present numerous security implications that must be addressed to ensure their safe and ethical deployment. Understanding these implications is crucial for developers, businesses, and policymakers to navigate the complex landscape of AI security.
Data Privacy and Confidentiality
One of the foremost security concerns with AI models is data privacy. AI systems often require large amounts of data to train effectively, and this data can include sensitive personal information. For example, healthcare AI systems might use patient records, while financial AI systems might utilize transaction histories. If these datasets are not properly anonymized, they can lead to privacy breaches.
Data breaches can occur due to hacking, inadequate encryption, or even insider threats. Once sensitive data is exposed, it can be misused for identity theft, financial fraud, or other malicious activities. Ensuring robust encryption, secure data storage, and strict access controls such as Zero Trust AI Access (ZTAI) are essential measures to mitigate these risks.
Model Theft and Intellectual Property
AI models themselves can be valuable intellectual property. Advanced models require significant resources to develop, including computational power, expertise, and time. As a result, they become attractive targets for theft. Adversaries may attempt to steal these models through cyberattacks, such as reverse engineering, where they use input-output pairs to reconstruct the model's internal structure.
Protecting AI models from theft involves implementing strong cybersecurity measures, such as secure coding practices, regular security audits, and using techniques like model watermarking, where unique, traceable signatures are embedded within the model.
Adversarial Attacks
AI models, particularly machine learning-based, can be vulnerable to adversarial attacks. These attacks involve feeding the model carefully crafted inputs designed to deceive it into making incorrect predictions or classifications. For instance, an adversarial attack on an image recognition system could make it misidentify objects by subtly altering the pixel values.
Adversarial attacks can have serious consequences, especially in critical systems like autonomous vehicles or medical diagnostics, where incorrect decisions can lead to accidents or misdiagnoses. Defending against these attacks requires developing robust models that can detect and resist adversarial inputs, employing techniques such as adversarial training and robust optimization.
Model Poisoning
Model poisoning, also known as data poisoning, involves corrupting the training data to influence the behavior of the AI model. Attackers can insert malicious data into the training set, causing the model to learn incorrect or harmful patterns. For example, in a spam detection system, an attacker might insert emails that are spam but labeled as non-spam, leading the model to incorrectly classify future spam emails.
To mitigate model poisoning, it's crucial to implement data validation and cleaning processes, as well as to use techniques like differential privacy, which ensures that a single data point does not significantly affect the model's output.
Model Inversion and Membership Inference
Model inversion attacks occur when an attacker uses the model's predictions to infer sensitive information about the training data. For example, an attacker could use an AI model trained on medical records to reconstruct patient information. Membership inference attacks are a related concern, where an attacker determines whether a particular data point was part of the training set.
These attacks highlight the need for privacy-preserving machine learning techniques. Federated learning, where models are trained locally on devices and only aggregated updates are shared, and techniques like differential privacy can help protect against these risks by reducing the amount of sensitive information exposed during model training and inference.
Bias and Fairness
AI models can inadvertently learn and perpetuate biases present in the training data. This can lead to discriminatory outcomes in applications such as hiring, lending, and law enforcement. Bias in AI models not only poses ethical concerns but can also result in legal and reputational risks for organizations.
Ensuring fairness in AI requires careful attention to the data used for training, including representative sampling and bias detection. Techniques like fairness-aware machine learning, which incorporates fairness constraints into the model training process, and regular audits for bias in AI systems are essential steps to address these issues.
Explainability and Accountability
AI models, particularly deep learning models, often operate as "black boxes," making it difficult to understand how they arrive at their decisions. This lack of explainability can be a significant security and ethical concern, especially in high-stakes domains like healthcare and finance, where understanding the rationale behind decisions is critical.
Improving the transparency and explainability of AI models involves developing methods for interpretable AI, where the decision-making process of the model can be understood and verified by humans. Techniques such as model-agnostic interpretability methods, which provide explanations without modifying the model, and inherently interpretable models are crucial for fostering trust and accountability in AI systems.
Ethical and Legal Implications
The deployment of AI models entails significant ethical and legal considerations. Ensuring ethical use of AI systems requires compliance with regulations, such as data protection laws like the GDPR, and adherence to ethical principles including fairness, accountability, and transparency.
Organizations must establish clear guidelines and frameworks for the ethical use of AI, including conducting regular impact assessments to identify and mitigate potential harms. Developing AI with ethical considerations in mind, known as ethical AI, is becoming increasingly important as AI systems play a more prominent role in society.
Conclusion
The security implications of working with AI models are multifaceted and complex. From data privacy and model theft to adversarial attacks and ethical considerations, there are numerous challenges that must be addressed to ensure the safe and responsible deployment of AI systems. Implementing robust security measures, adopting privacy-preserving techniques, and ensuring fairness and transparency are crucial steps in mitigating these risks. As AI technology continues to evolve, ongoing research and collaboration between developers, policymakers, and other stakeholders will be essential to navigate the dynamic landscape of AI security and harness the full potential of AI in a safe and ethical manner.